Andriyan Bayu Suksmono
STEI-ITB
Tati Latifah Mengko
STEI-ITB
Donny Danudirdjo
STEI-ITB
Suci Aulia
STEI-ITB
Rika Sustika
STEI-ITB
A Novel Digitized Microscopic Images of ZN-Stained Sputum Smear and Its Classification
Based on IUATLD Grades
Microscopic detection of acid-fast bacilli (AFB) from mycobacterium tuberculosis (MTB) in Ziehl-Neelsen (ZN)-stained sputum samples is a crucial step in the detection of TB (tuberculosis) disease. Pathologists encounter many challenges that may result in incorrect diagnoses, such as the heterogeneous shape and irregular appearance of MTB, low-quality ZN staining, and errors in in scanning each of the field of view (FoV) using a conventional microscope. Additionally, multiple manual observations may cause fatigue that leads to human error. Nevertheless, the implementation of computer-aided diagnosis (CAD) systems for TB diagnosis remains an area of ongoing research and development owing to the lack of microscopic image datasets of sputum samples, which represent whole-slide imaging (WSI) that follows the WHO (World Health Organization) regulations. To address this issue, Our study provides new insights into various areas that have not been covered in previous research. First, we created a new dataset of TB sputum sample images, namely the Microscopic Imaging Database of Tuberculosis Indonesia (MIDTI). MIDTI was categorized based on the IUATLD grade and digitized system, according to the WHO standard. The MIDTI images had dimensions of 3072 × 2304 pixels in (. jpg) format, as detailed in Table 1.

Second, our study proposed CAD for TB based on sputum examination using a method that is based on the YOLOv7_RepVGG.
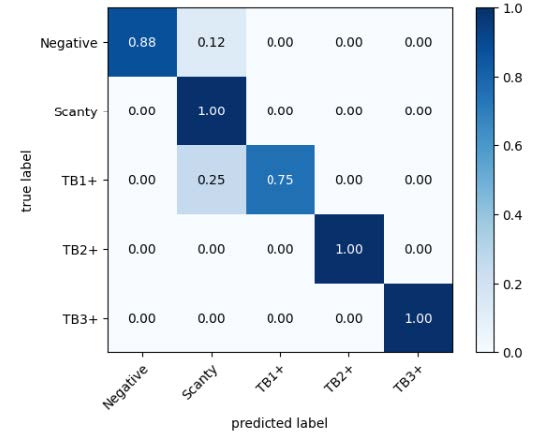
This study classifies sputum smears into IUATLD grades: negative, scanty, TB1+, TB2+, and TB3+, which have never been revealed in previous studies. . Fig.1 shows the performance results of our proposed model using YOLOv7_RepVGG for the five-class IUATLD. Fig.2 shows the visualization of the proposed model that detects AFB per FoV.

Publication:
S. Aulia, A. B. Suksmono, T. R. Mengko and B. Alisjahbana, “A Novel Digitized Microscopic Images of ZN-Stained Sputum Smear and Its Classification Based on IUATLD Grades,” in IEEE Access, vol. 12, pp. 51364-51380, 2024, doi: 10.1109/ACCESS.2024.3386208.
Residual Double Attention Network for Remote Sensing Image Pansharpening
Introduction
Pansharpening aims to obtain high spatial resolution multispectral (MS) images by fusing the spatial and spectral information in low spatial resolution (LR) MS and panchromatic (PAN) images. In the last few years, many studies with deeper and wider convolutional neural networks (CNN) have improved the fusion quality. Nevertheless, a few issues associated with previous CNN-based methods were introduced during fusion.
To enhance the spatial and spectral information in the fused image, we proposed a pansharpening method based on the attention mechanism. This approach can learn correlations between channels and resulted significant improvements in image classification, object detection, and super-resolution tasks. Recently, it has been proven to be effective in improving pansharpening performance.
Method
This study proposed a pansharpening method that combined channel and spatial attention mechanism networks, namely the residual double-attention network (RDAN). Fig. 1 shows the proposed architecture. In the preprocessing step, the observed MS image patch was upsampled to the PAN size using a polynomial interpolator. The upsampled MS image and PAN image were used as the inputs for the RDAN network. The proposed network consists of three main parts: shallow feature extraction (SFE) unit, double-attention feature fusion (DAFF) module, and image reconstruction (IR) part. The SFE unit was responsible for extracting the spatial and spectral features from the PAN and MS images. Spatial and spectral information were combined using the DAFF module. Finally, the pansharpened MS image were reconstructed using the IR part.

Discussion & Result
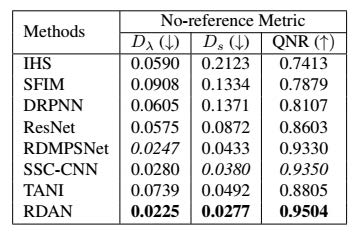
Non-reference metrics were used to examine the pansharpening performance of the full-resolution experiments. 𝐷𝜆 was used to evaluate spectral distortions, 𝐷𝑠 was used to assess the spatial distortions, and QNR was used as a comprehensive metric. The table result above shows that in the full-resolution condition, the proposed method (RDAN) performed better than the other methods. These results verify that the proposed approach improved the spectral and spatial information in the MS images better than the other methods.
Conclusion
The RDAN combine a channel and spatial attention module in residual structure. The experimental results verifies that exploiting both is superior to using only a single type of attention. The residual (skip) connections within the low and high layers allow lower-level information to propagate to the higher layer, helping the network to preserve information that would have been lost through the training process with many layers. The proposed method has been proven to be effective for pansharpening task.
Publication:
R. Sustika, A. B. Suksmono, D. Danudirdjo and K. Wikantika, “Remote Sensing Image Pansharpening Using Deep Internal Learning With Residual Double-Attention Network,” in IEEE Access, vol. 12, pp. 162285-162298, 2024