Dr. Ir. Nanang Hariyanto, M.T.
Sekolah Teknik Elektro dan Informatika
Dr. Fathin Saifur Rahman, S.T., M.T.
Sekolah Teknik Elektro dan Informatika
The intermittency generated by variable renewable energy (VRE) from photovoltaic power plants (PVPPs) will negatively affect frequency stability, particularly during their high penetrations in the system. One of the methods to mitigate the impact of the VRE intermittency is by using photovoltaic (PV) curtailment. However, performing PV curtailment will result in unused power from PVPPs. Therefore, to maximize the utilization of output power from PVPP while maintaining frequency stability, it is important to determine the appropriate level of curtailed power from PVPPs.
In this study, very short-term forecasting is utilized to predict the timing and magnitude of upcoming intermittency. The forecasting predicts PV power output for the next 3, 5, and 7 minutes. Historical data is used to predict PV power fluctuations. By comparing various machine learning (ML) models, the most suitable model is identified based on several evaluation criteria. To further maximize the utilization of output power from PVPPs during curtailment, based on the forecasting result, the maximum power point (MPP) of the PVPP during curtailment can be predicted, in which event-triggering virtual inertia (ETVI) is then can be implemented based on the curtailed power. This will effectively change the curtailed PV power which was previously unused, into a reserve power capable of supporting the frequency stability of the system in the case of system contingency.
Based on the performed simulations, by utilizing the proposed method, the utilization of output power from PVPPs can be maximized without sacrificing the frequency stability of the system while also providing means of frequency support to enhance frequency stability during contingency.
Keyword: Event-triggering virtual inertia, photovoltaic (PV) curtailment, renewable energy, very short-term forecasting.
Introduction
The transition to renewable energy in Indonesia faces challenges, including the need to add nearly 60 GWp of photovoltaic (PV) capacity to achieve net-zero emission. It will affect system stability, as increased PV integration reduces system strength and inertia, making frequency control more challenging. Intermittency in PVPPs can be addressed through curtailment, which manages excess generation and supports frequency stability. However, curtailment leads to unused PV power, necessitating an optimal approach to balance frequency stability and power utilization. This study proposes methods for determining the appropriate curtailment level using historical data and forecasting techniques, alongside developing a power frequency correlation function to enhance system reliability.
Very short-term forecasting models based on machine learning (ML) such as Adaboost, Random Forest, and Neural Networks are utilized to predict PV power output for the next few minutes, improving curtailment planning and frequency support. The paper also introduces event-triggering virtual inertia (ETVI), enabling curtailed PV power to act as virtual inertia, enhancing system stability. Key contributions include identifying the best ML model for PV forecasting, estimating the maximum power point (MPP) during curtailment, and optimizing PVPP output for ETVI to improve system
Research Method
In this paper, a step-by-step approach consisting of three main steps is employed as follows and summarized in Fig. 1.
• Estimation of PV curtailment based on very short-term forecasting.
• Maximum power point (MPP) forecast of PVPPs during curtailment.
• The utilization of PV curtailment as event-triggering virtual inertia (ETVI).
The maximum power point (MPP) of PV power plants (PVPPs) is forecasted using historical data and machine learning (ML) methodologies implemented through the Orange application. Data preprocessing, including outlier removal, data filtering, and normalization, ensures high-quality input for analysis. The Pearson correlation coefficient is used to identify strong relationships between variables like irradiation, temperature, and wind speed with generation, helping select the most influential features for modeling. ML techniques such as Tree, Random Forest, and Neural Networks are evaluated through cross-validation to determine the most accurate model for predicting MPP, especially during curtailment scenarios.
Very short-term forecasting predicts PV power fluctuations within minutes, enabling proactive curtailment measures. Forecasting models are compared using metrics like RMSE, MAPE, and R-squared to identify the best-performing approach. Estimated curtailed PV power is then utilized as event-triggering virtual inertia (ETVI), enhancing grid stability. By simulating the contribution of curtailed PV power as spinning reserves during disturbances, the study demonstrates how PVPPs can support system reliability through optimized curtailment and frequency response.
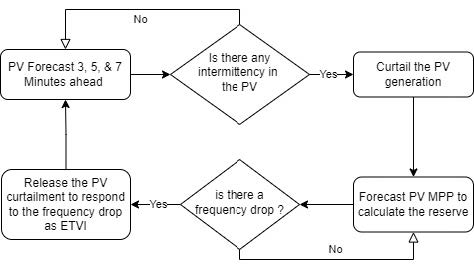
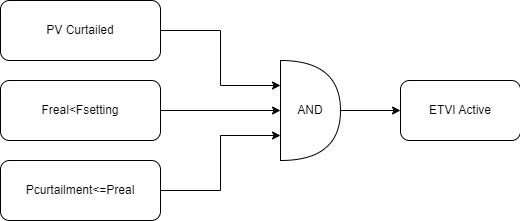
Discussion & Result
Forecasting Result: The study applies very short-term forecasting to predict PV power fluctuations within a 7-minute window. Using ML models such as AdaBoost, Random Forest, and Neural Networks, the AdaBoost model showed the best performance for 3-, 5-, and 7-minute forecasts with real-time data (T-0). Metrics like MAPE were kept below 10%, indicating reliable accuracy. However, limitations emerged due to sensor constraints and data lags, which sometimes delayed predictions or caused forecast failures. Despite these challenges, the forecasting method effectively identifies optimal curtailment levels and reserve capacities for enhancing system stability.
ETVI Implementation: The curtailed PV power is utilized as ETVI to support frequency stability during grid disturbances. Simulations show that activating ETVI at 49.6 Hz yields the best results, preventing under-frequency relay (UFR) activation and maintaining stable voltage and rotor angle responses. By releasing active power from PV curtailment, the system compensates for frequency deviations and stabilizes voltage conditions, particularly near disturbance locations. This approach maximizes the value of curtailed PV power by transforming it into a spinning reserve resource.
Overall Impacts: Combining forecasting and ETVI utilization enhances the reliability of PV-integrated grids. Forecasting predicts intermittency patterns and curtailment needs, while ETVI ensures frequency and voltage stability during contingencies. Together, these methods enable efficient use of curtailed PV power, improving grid resilience without compromising system operations. By addressing key challenges in renewable energy integration, the proposed methods pave the way for more stable and reliable grid systems.
Conclusion
In this paper, it is demonstrated that very short-term forecasting can serve as a guide to determine when and to what extent PV power reduction due to intermittency may occur. Despite its drawback of lagging in predicting reduction patterns, the magnitude of power reduction can be forecasted, enabling the identification of necessary curtailment times. Forecasting the maximum power point (MPP) is essential to ascertain the reserves available during PV curtailment. Utilizing these reserves during PV curtailment by activating the event-triggering virtual inertia (ETVI) scheme enables the utilization of curtailed PV power and leads to improved system stability. Based on the simulation results, the most ideal setting for ETVI activation occurs when the frequency reaches 49.6 Hz. Utilizing curtailed PV power can contribute to limiting frequency deviation and prevent the first stage of under-frequency relay (UFR) operation.